FINHOUND AI
People make mistakes
Messy and complex data
Labour intensive routine
Legal risks and reputational losses
FINHOUND is here to help
MassChallenge 2024FinTech Cohort
FinHound was selected to participate in the MassChallenge 2024 FinTech Program. FinHound was one of over 200 startup applications for this year’s program.
During our partnership with MassMutual, we are streamlining their internal labour-intensive processes saving time and money for the insurance company.
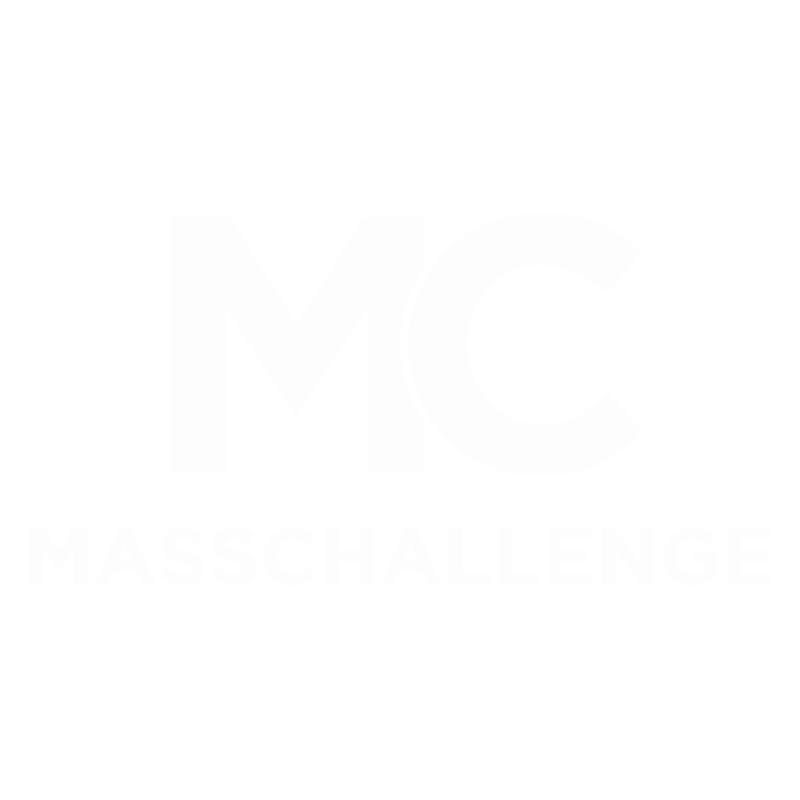

How does it work?
Use case: Finalizing Reports for Audit Firms
Outstanding OCR
FinHound accurately recognises and extracts data from PDFs/scanned documents of any complexity.
Our Optical Recognition Abstract Layer (ORAL) is based on DETR (End-to-End Object Detection with Transformers), state-of-the-art neural networks. ORAL’s features are fine-tuned for tables and various page layouts, improving the precision and versatility of data extraction.
Matching data
FinHound compares auditing reports across different periods.
Utilizing its own lightweight and efficient Vector database, FinHound analyzes and searches for matches across different documents.
100+ Automatic Checks
FinHound indicates exactly where inconsistencies, typos and errors are found, pinpointing their location and origin.
Finhound's algorithm checks the consistency of different financial entities taking into account the contextual factors, such as the corresponding timeframes and dependency relationships, ensuring a more comprehensive analysis of financial data.
Natural Language Processing (NLP)
FinHound’s NLP models detect characteristic financial entities of every company and can be easily adjusted for various industries.
Leveraging the Natural Language Toolkit (NLTK), our system establishes a foundational comprehension of document context, laying the groundwork for subsequent data processing stages.
Context Understanding
FinHound’s models do not simply sum the numbers within a table but understand the context of the whole document and catch cross-reference mistakes.
Utilizing neural networks with Transformers and attention mechanisms, our platform enhances verification of contextual details in the data.
Convenient Summary
FinHound generates an annotated summary based on the original documents.
During in-depth sessions with potential clients, we establish their needs and customize the summary in order to streamline their process and save them time.
Watch video
Book a demo
F.A.Q
At Finhound, we specialize in streamlining routine labor-intensive processes using OCR, AI, and machine learning. Our solutions focus on optimizing tasks in areas such as:
- Data Entry: Using OCR to automate manual data entry tasks for increased accuracy and efficiency.
- Document Analysis: Utilizing AI for seamless extraction of information from various document formats.
- Data Integration: Streamlining the integration of data across different sources for a unified view.
- Workflow Automation: Optimizing repetitive tasks through advanced AI and machine learning algorithms.
Utilizing DETR neural networks, FinHound enhances data extraction precision.
By combining NLTK, neural networks with Transformers and attention mechanisms, along with FinHound's proprietary vector database, our AI-platform offers a flexible and transparent solution to verify and enrich data.
FinHound excels in understanding context and identifying cross-reference mistakes within financial/etc statements.
Utilizing DETR neural networks, FinHound enhances data extraction precision.
By combining NLTK, neural networks with Transformers and attention mechanisms, along with FinHound's proprietary vector database, our AI-platform offers a flexible and transparent solution to verify and enrich data.